2. what other tools might be used to assess mrs. baxter’s nutritional status?
- Inquiry article
- Open Access
- Published:
Cess of nutritional status using anthropometric variables by multivariate analysis
BMC Public Health volume xix, Article number:1045 (2019) Cite this article
Abstract
Background
Undernutrition is a serious health problem and highly prevalent in developing countries. In that location is no every bit such confirmatory examination to measure undernutrition. The objective of the present written report is to determine a new Composite Score using anthropometric measurements. Composite Score was then compared with other methods like torso mass index (BMI) and mid-upper arm circumference (MUAC) classification, to test the significance of the method.
Methods
Anthropometric data were collected from 780 adult Oraon (Male person = 387, Female = 393) labourers of Alipurduar district of West Bengal, India, post-obit standard instruments, and protocols. Nutritional status of the study participants were assessed by conventional methods, BMI and MUAC. Confirmatory gene analysis was carried out to reduce 12 anthropometric variables into a unmarried Composite Score (C) and classification of nutritional status was done on the basis of the score. Furthermore, all the methods (BMI, MUAC and C) were compared and discriminant office analysis was adopted to notice out the per centum of correctly classified individuals by each of the 3 methods.
Issue
The frequency of undernutrition was 45.ix% according to BMI category, 56.seven% co-ordinate to MUAC category and 51.eight% according to newly computed Blended Score. Further analysis showed that Composite Score has a college strength of right nomenclature (98.7%), compared to BMI (95.nine%) and MUAC (96.2%).
Conclusion
Therefore, anthropometric measurements can be used to identify nutritional condition in the population more correctly by computing Composite Score of the measurements and information technology is a not-invasive and relatively correct manner of identification.
Background
Human being torso needs a proper nutrition through well counterbalanced diet to fulfill torso requirements and to maintain basic body physiology. Improper nutrition leads to the consumption of excess calorie (over-nutrition) or insufficient supply of one or more essential nutrients (under-nutrition). Over-nutrition is a threat that increases body weight and causes several not-infectious disease. On the other, undernutrition, acquired due to the insufficient intake of energy and nutrients, is a serious health trouble for the economically backward, developing countries like Bharat [i]. It causes nutrition related complications, different deficiency diseases and even death by decreasing body immunity [ii,3,4,five]. The curt-term effect of undernutrition is weakness and recurring illness. Whereas, in the long run it hampers all vital functions causing low weight, growth retardation of children and boyish, decreased immunity leading to recurring infections [six], occurrence of chronic diseases similar diabetes mellitus, hypertension, and coronary heart diseases in later adult life [7] and impaired mental development [8]. Furthermore, in women, undernutrition may cause obstetric complications leading to maternal and infant mortality and increases the probability to requite low nascency weight babies and thus leading to the undernutrition cycle start again, spanning several generations [3, 9,x,11]. Besides, a chronic undernutrition also causes a reduced work capacity and ability to sustain economically productive work resulting in low income [12, 13]. Therefore, undernutrition is a critical burden and curse for the development of human being likewise as for the society.
The main causes of undernutrition can be broadly classified equally biological, behavioral and sociological factors [vi, three]. The biological causes may be infectious diseases like HIV/AIDS, TB etc. and also helminthes infestation which decreases intestinal nutrient absorption and thus developing poor diet. Behavioral factors include insufficient access to nutrient, inadequate or inappropriate noesis, exercise and sanitation. The major social risk factors are political situation, lack of didactics and economic inequality [14,15,sixteen]. Cultural influences on food habits along with several religious taboos and social customs may also cause nutritional deficiency [17,18,19].
Thus, undernutrition is a status of poor nutritional status resulting from reduced food intake or impaired metabolism and evaluation of nutritional condition is necessary to determine the severity of undernutrition. As there is no objective test to mensurate nutritional status, therefore numerous screening methods accept been adult to make up one's mind the nutritional status of individual: (i) assessing clinical signs and symptoms, (ii) biochemical indicators (iii) dietary survey and (iv) anthropometric measurements. Cess of clinical signs and symptoms need proper knowledge for evaluation, whereas biochemical indicators are relatively expensive and time consuming to perform in community level. On the other, dietary survey can give an idea of daily free energy intake simply there may have chances of misreporting and also need nutrient consumption data of several days to obtain the gauge of usual diet.
Anthropometry has a long tradition of assessing nutritional and health status of adults as this is an inexpensive, non-invasive method that provides detailed information on different components of body construction, specially muscular and fatty components [20, 21]. Moreover, anthropometric measurements are highly sensitive to the broad spectrum of nutritional status, whereas biochemical and clinical indicators are useful but at extremes of malnutrition. Among the widely used anthropometric measurements, body mass index (BMI) and mid-upper-arm-circumference (MUAC) are most significant and reliable.
BMI (Body mass alphabetize) is more often than not considered as a adept indicator and used for the cess of chronic free energy deficiency of adults, especially in developing countries [22,23,24]. It is highly correlated with fat and fat-gratuitous mass so the protein and fat reserves of torso tin can exist estimated. In normal adults the ratio is approximately constant, and a person with a low BMI is underweight for his/her height.
Nevertheless, at that place are some difficulties associated with the sole use of BMI, for example the ratio of sitting summit to standing height or cormic alphabetize can influence BMI [25]. Cormic alphabetize varies both between populations and within populations [26]. So, without the correction by cormic index equally a correction factor, the sensitivity and specificity of BMI every bit an indicator of diet may be low. Historic period is another factor that may alter the functional significance of BMI at different ages; considering adults tend to loose fat costless mass and increase fat mass with increasing age [26,27,28]. Oedema can also touch the significance of BMI. Adults may develop oedema when severely undernourished, which artificially increases an individual's weight resulting in BMI actualization more normal than the actual value [29]. Moreover, the universal cut-off of the BMI cannot be applicable across different populations [thirty]. Then, these inabilities limit the usefulness of BMI every bit an authentic screening tool to assess adult undernutrition.
On the other, MUAC (Mid-upper arm circumference) is some other important indicator for elementary screening of adult nutritional status, specifically in developing countries [31, 32]. The measurement requires fewer apparatus and easy to perform even on the virtually debilitated individuals. It is independent of height and indicates the arm muscle and sub-cutaneous fatty; both being of import determinants of survival in starvation. Though classification of undernutrition according to the MUAC category is more appropriate than BMI category, but is not completely mistake gratis. Bereft data are bachelor correlating MUAC with undernutrition and other functional measures in adults, beyond unlike ethnic and population groups. Furthermore, the use of MUAC in adults may exist affected by the redistribution of subcutaneous fat towards central areas of the body during crumbling [33]. Therefore, age-specific cut-off points of MUAC may exist required. MUAC is as well very sensitive towards intra- and inter- observer errors.
In view of the in a higher place, a more than authentic and population specific method is necessary to appraise the nutritional status of the population using anthropometric measurements. Present article documents the development of the method with specific statistical tools.
Methods
Study population
Anthropometric data were collected as part of an ongoing bio-medical project on Oraon labourers of Alipurduar district of West Bengal, India. Data includes 780 (Male 387, Female 393) adults from ii occupational subgroups, i engaged in agriculture and other in tea garden, both having similar socio-economical status and living condition. The study was canonical past the Ethical Committee of Indian Statistical Plant, Kolkata and was performed with the prior written consent from the participants. No statistical sampling was followed, because any kind of option within the population would have raised suspicion in the minds of the people studied, regarding the purpose of the study. However, the participants were chosen without whatsoever conscious bias; actually the participants who could be persuaded to participate in the study and volunteered for participation in the study were included in the sample. Studies on the nutritional condition of the indigenous population have an of import significance in context of wellness planning. Thus, the present anthropometric measurements were collected from one of such indigenous group, Oraon in the Dooars foothill region of West Bengal.
Anthropometric measurements
Anthropometric measurements were obtained following standard protocol and instrument [34]. These were two length measurements i.due east. height (Ht.) and sitting height (SHt.), measured (0.1 cm) past Martin'southward anthropometric rod (GPM, Switzerland). Weight (Wt.) was measured (0.i kg) with an electronic scale (Omron HBF-375 Karada Scan, Nihon). Five circumferences i.east. dogie (CC), mid upper arm (MUAC), chest (CCN), waist (WC) and hip (HC) were measured past measuring tape. Five skinfolds i.e. calf (CSK), biceps (BSK), triceps (TSK), sub-scapular (SBSK) and supra-iliac (SISK) were measured (0.1 mm) on the left side of the body by Holtain skinfold caliper. Four diameters i.e. bi-epicondylar diameter of humerus (BDH), bi-condylar diameter of femur (BDF), bi-acromial diameter (BAD) and bi-iliac diameter (BID) were measured (0.1 cm) past sliding caliper and spreading caliper (GPM, Switzerland).
Nutritional status
Nutritional status of the study population was assessed in terms of the two conventional methods i.e. BMI (Body mass alphabetize) [24] and MUAC (Mid-upper arm circumference) [32].
(BMI) has been calculated using the formula: \( BMI=\frac{Weight\ (Kg.)}{Height^2\ \left({m}^ii\correct)} \)
The criteria used for the classification of the nutritional condition have been described in Table 1.
As the hateful of BMI and MUAC of the studied sample were 18.99 and 22.20 cm, so for the analytical purpose, nutritional condition was classified into 2 categories; (a) Chronic energy deficiency or undernutrition and (b) normal.
Statistical assay
Initially, sixteen anthropometric measurements, which have previously been used for nutritional assessment, were used in the analyses. Amidst them twelve variables were found meaning correlation with summit, weight and MUAC. These were used for farther statistical testing. Furthermore, by elimination method information technology was verified that the selected 12 variables can describe the highest variance of the newly computed variable. Thus these twelve variables created a single score variable that helped significantly for the cess of nutritional status.
Descriptive statistics of all the variables were calculated. Then Starting time Social club Confirmatory Factor Analysis (CFA) and Discriminant Part Assay were performed. All of the statistical analysis was carried out using PASW, version 18.0 (SPSS Inc., Chicago, IL, United states of america) and STATA, version 13.0 (STATA Corp, The states).
Confirmatory gene analysis
Confirmatory factor analysis (CFA) helps to examination the hypothesis that a relationship between observed variables and their underlying latent construct exists. This analysis is primarily a theory driven statistical data reduction technique used to explicate covariance among different observed random variables and thereby reducing large number of variables to parsimonious and meaningful groups of underlying unobserved variables named factors [35]. The analysis gives a path diagram for the measurement model in STATA. In this written report, in that location were twelve anthropometric variables (observed variables) in the rectangular boxes which accept a commonality or shared variance or covariance. This covariance corresponds to the latent factor or latent variable. The observed anthropometric variables are related to the latent variable through gene loadings which are fundamentally regression coefficients. A part of variance of the indicators (observed variables) that cannot be explained by the latent factor is termed equally measurement mistake of the model and therefore unique to each observed variable. The latent factor, observed variables and the measurement errors together describe a linear equation and can be expressed in matrix grade as:
$$ Ten={\Lambda}_x\xi +\varepsilon, $$
Where Ten is a cavalcade vector of 12 standardized anthropometric variables, Λ x is a 12 × ane matrix of coefficients relating each variable to its latent factor, ξ is the latent variable and ε is the measurement errors, which is a 12 × one matrix.
\( X=\left[\brainstorm{array}{ccc}{Ten}_1& =& Ht.\\ {}{10}_2& =& Wt.\\ {}{X}_3& =& MUAC\\ {}{X}_4& =& CC\\ {}{X}_5& =& CC Northward\\ {}{X}_6& =& WC\\ {}{X}_7& =& HC\\ {}{X}_8& =& BSK\\ {}{X}_9& =& TSK\\ {}{X}_{10}& =& CSK\\ {}{Ten}_{eleven}& =& SBSK\\ {}{X}_{12}& =& SISK\terminate{array}\correct],\kern0.5em {\Lambda}_x=\left[\brainstorm{array}{c}{\lambda}_1\\ {}{\lambda}_2\\ {}{\lambda}_3\\ {}{\lambda}_4\\ {}{\lambda}_5\\ {}{\lambda}_6\\ {}{\lambda}_7\\ {}{\lambda}_8\\ {}{\lambda}_9\\ {}{\lambda}_{10}\\ {}{\lambda}_{11}\\ {}{\lambda}_{12}\cease{array}\right],\in =\left[\begin{assortment}{c}{\in}_1\\ {}{\in}_2\\ {}{\in}_3\\ {}{\in}_4\\ {}{\in}_5\\ {}{\in}_6\\ {}{\in}_7\\ {}{\in}_8\\ {}{\in}_9\\ {}{\in}_{10}\\ {}{\in}_{11}\\ {}{\in}_{12}\end{array}\right],\kern0.5em \)
Thus latent variable was calculated from each linear equation for each private and was termed every bit Composite Score. Afterward Composite Score was used as an alternative variable instead of the 12 anthropometric variables (observed variables) and a new classification was developed on the footing of this score. Negative Composite Scores were considered every bit undernutrition and positive Scores equally normal nutritional status.
Discriminant office analysis
Discriminant function analysis is primarily a multivariate test to observe the differences between groups. This is the contrary of MANOVA, where the independent variables are the continuous predictors and dependent variables are the groups [36].
The analysis can exist split into 2-steps- (a) testing significance of a set of discriminant functions, and, (b) classification. In the written report, 2d step of the analysis was used for the classification of nutritional status in view of the equations created in the analysis. Computationally a canonical correlation analysis was performed and that determined the successive functions and canonical roots. Nomenclature was then possible from the canonical functions. Individuals were classified in the groups in which they had the highest nomenclature scores [37]. This analysis further provided a per centum of overall right classification.
Event
Tabular array 2 depicts the descriptive statistics of age, selected anthropometric traits and body mass alphabetize of the study population of either sex. It was observed that except the mean skinfold values, all other mean values were college in males than females which indicate the poor nutritional status of women labourers of the present study grouping.
Tabular array 3 is the tabular course of the path diagram (Fig. i), information technology depicts the values of coefficients and measurement errors of the model, which were used to compute the Composite Score in the analysis.
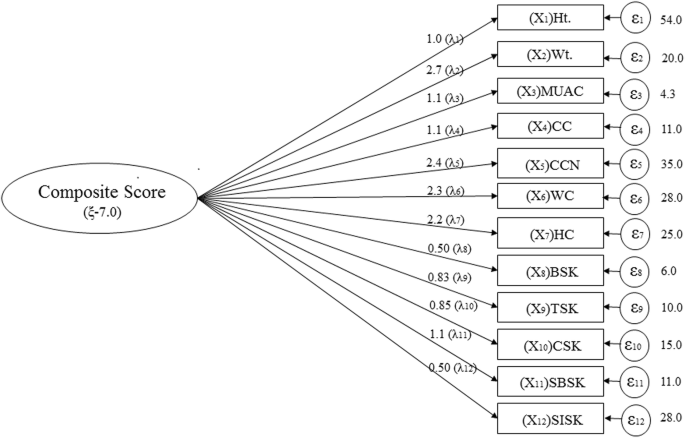
Path diagram of the Measurement Model of Confirmatory cistron analysis. Describes the path diagram of measurement model of the confirmatory factor analysis. The variance of Composite Score (ξ) was 7.0. The values of the respective cistron loadings (λi) were mentioned and the measurement error (ɛi) of the model for each respective observed variable was likewise calculated as shown
Table four depicts the frequencies and percentages of individuals categorized under undernutrition and normal category considering BMI, MUAC and Blended Score of the present analyses. Information technology was observed that according to BMI forty.i% males and 51.7% females were categorized as undernutrition. Whereas the per centum (Male = 42.9% & Female = lxx.2%) of undernutrition increased using MUAC scale. However, Composite Score classified 38.2% males and 65.i% females nether undernutrition. The differences of frequencies (undernutrition and normal) classified past BMI and Composite Score were institute pregnant (z-test for equality of proportion was done) simply for females.
Table v gives the values of coefficients of discriminant function analysis and also assesses how well the Fisher'due south classification role coefficients were classified between the groups. The coefficients were used to construct a discriminant office for each grouping, i.e. under nutrition and normal.
For Blended Score
$$ \mathrm{Undernutrition}=-1.395+\left(-0.741\correct)\ast Composite\ Score $$
(1)
$$ \mathrm{Normal}=-ane.507+0.799\ast Composite\ Score $$
(2)
Equations (1) and (2) accept to be calculated for each case to become the undernutrition or normal value and the instance volition be classified for which computed value will exist higher. For example, if eq. (two) gives the higher value for a particular example, and then the instance will exist classified every bit normal.
Table 6 describes the result of discriminant factor analysis. It was observed that according to the BMI classification, 7.6% were misclassified as normal. Co-ordinate to MUAC category, vi.viii% was misclassified every bit undernutrition. For Composite Score misclassification were but 2.7, who were wrongly classified equally normal. Furthermore, it was observed that according to the BMI calibration the overall correct prediction was 95.ix%, and by MUAC calibration information technology was 96.2%. In comparison with BMI and MUAC classification, the newly computed Blended Score has the higher strength (98.7%) of classifying overall nutritional status.
Discussion
The present study tries to detect out the about precise way of assessing nutritional condition. Anthropometry has long been used as indicator of nutritional status considering it is non-invasive and less expensive. Anthropometric measurements assistance in calculating both BMI and MUAC, which provide a simple and convenient value for assessing nutritional condition. Nutritional assessments in rural population usually rely on BMI and MUAC, every bit it does not require much instruments, fourth dimension and efficiency; but take independent limitations. For example, individuals with ectomorphic somatotype may exist misclassified as undernourished with BMI classification. Once again, human body has bilateral disproportion; therefore, taking MUAC measurement on 1 side may provide erroneous cess. On the other, variation of MUAC is very high depending on the concrete activity and food intake of the individual. Moreover, both BMI and MUAC exercise not have population specific cut-off values. Therefore, there are every chances of misclassifying nutritional status of individuals.
The endeavour of the present study was to develop a better and rigorous tool that can easily identify nutritional status. A number of anthropometric measurements were used to construct a new method for assessing nutritional status. All the anthropometric traits were tested for its clan to top, weight and MUAC, even so, found 12 anthropometric traits that have precise and expert clan with height, weight and MUAC. Those 12 anthropometric traits represented into i Composite Score by confirmatory factor analysis (Fig. i). Later, nomenclature was done on the ground of Blended Score and was compared with other two classification categories (BMI and MUAC) past discriminant function analysis to find out which 1 gives the all-time classification.
A separate equation for undernutrition and normal was obtained from discriminant office assay (Table 5) for BMI, MUAC and Composite Score which was used to predict the corresponding frequencies. In comparison with BMI and MUAC category, information technology was observed (Tabular array four) that frequency of undernutrition and normal (well-nourished) individuals significantly vary when categorized with Composite Score and the difference in frequency was found higher in instance of females than males. It may be considering the visceral redistribution of fat predominantly affects females [38] thereby causing differences in fat patterning between sexes [39]. Therefore disparity in measurements is always prominent in females. Then the same analysis was also used to test the strength of the classification category on the basis of observed and predicted values (Tabular array six). It was observed that the newly computed Composite Score qualifies for the highest forcefulness (98.7%) to identify the individuals in the specific category followed by MUAC (96.ii%) and BMI (95.nine%). This may exist because; as the newly computed Composite Score is a multidimensional method of nutritional cess therefore it may have maximum chances of correct classification.
The assessment of nutritional status by anthropometry was previously done primarily on the basis of BMI or MUAC [31, twoscore, xx, 41]. Some studies also used skinfold measurement similar BSK, TSK and CSK [42, 43, 32, 44] for the nutritional cess. Waist circumference and waist-hip ratio was also used for nutritional assessment [45, 46]. Just there is hardly any study that take used the linear measurements like Ht., circumferences similar MUAC, CC, CCN, WC and HC, skinfolds like BSK, TSK, CSK, SBSK, SISK and Wt. to predict nutritional condition or accept been tried to group those. To our knowledge, it is hard to find comparable literatures on this issue because it is a new one.
Moreover, the cut-offs of all the nutritional assessment tools with anthropometric traits have aforementioned values, irrespective of ethnicity. Developed individuals of different ethnic backgrounds display differences in torso shape and cormic index. Studies found a higher pct of body fat at lower BMI in example of Asians [47]. So, it may be inappropriate to compare unlike population with a single universal reference value.
Simply in calculating the Composite Score, the values of anthropometric data are being used of the study population, and so the classification category is more specific for that written report group. Finally, it is believed that the newly developed method volition be useful in identifying nutritional status of the individuals of a particular study group more correctly.
Limitations
The nowadays study tried to appraise the nutritional status of a item ethnic group with selected anthropometric measurements past computing Composite Score. There are few limitations also in calculating such Composite Score; it needs some statistical computation which may not always exist viable in the field situation. Moreover, the method is applicable to identify only the nether-nourished and normal individuals, not the obese individuals. In the present data, every bit in that location were no obese individuals, and then this method has been applied successfully. Further study in unlike population with more sample size is needed to allocate the nutritional status for 3 categories post-obit the nowadays Blended Score method.
Determination
The adverse outcome of undernutrition on health, development and economic productivity is well established. It needs proper and accurate identification to get the idea of severity to address the issue. The newly computed Composite Score can predict the nutritional status more accurately than BMI and MUAC. Moreover, information technology will requite population based cut-off values which will lower the probability of mis-classification. Lastly, it is expected that scientific ventures volition proceed to develop such scores with the information of other population groups and information technology volition provide a comprehensive agreement over this newly adult method.
Abbreviations
- BAD:
-
Bi-acromial diameter
- BDF:
-
Bi-condylar diameter of femur
- BDH:
-
Bi-epicondylar diameter of humerus
- BID:
-
Bi-iliac bore
- BMI:
-
Body mass index
- BSK:
-
Biceps skinfold
- CC:
-
Calf circumference
- CCN:
-
Chest circumference (Normal)
- CFA:
-
Confirmatory factor analysis
- CSK:
-
Dogie skinfold
- HC:
-
Hip circumference
- Ht:
-
Top
- MUAC:
-
Mid upper arm circumference
- SBSK:
-
Sub-scapular skinfold
- SHt:
-
Sitting top
- SISK:
-
Supra-iliac skinfold
- TSK:
-
Triceps skinfold
- WC:
-
Waist circumference
- Wt:
-
Weight
References
-
Onis Doc, Monteiro C, Akre J, Clugston M. The worldwide magnitude of protein-energy malnutrition: an overview from the WHO global database on kid growth. Bull World Wellness Organ. 1993;71:703–12.
-
Schofield C, Ashworth A. Why have mortality rates for severe malnutrition remained so high? Bull World Wellness Organ. 1996;74:223–nine.
-
Maleta K. Undernutrition. Malawi Med J. 2006;18:189–205.
-
Every Adult female every kid. The global strategy for women'due south, children'due south and adolescents' health (2016–2030). 2005. http://www.who.int/life-form/partners/global-strategy/globalstrategyreport2016-2030-lowres.pdf. Accessed 12 Apr 2018.
-
Kuruvilla South, Bustreo F, Kuo T, Mishra CK, Taylor 1000, Fogstad H, et al. The global strategy for women's, children'due south and adolescents' health (2016-2030): a roadmap based on evidence and country experience. Bull World Wellness Organ. 2016;94:398–400.
-
Muller O, Krawinkel M. Malnutrition and health in developing countries. CMAJ. 2005;173:279–86.
-
Hoet JJ. The role of fetal and infant growth and nutrition in the causality of diabetes and cardiovascular affliction in later life. SCN News. 1997;fourteen:10–3.
-
Martins VJ. Toledo Florencio TM, Grillo LP, do Carmo P Franco Grand, Martins PA, Clemente AP et al. long-lasting effects of undernutrition. Int J Environ Res Public Health. 2011;8:1817–46.
-
Rotimi C, Okosun I, Johnson L, Owoaje Due east, Lawoyin T, Asuzu M, et al. The distribution and mortality impact of chronic energy deficiency amid adult Nigerian men and women. Eur J Clin Nutr. 1999;53:734–9.
-
Allen LH, Lungaho MS, Shaheen Thousand, Harrison GG, Neumann C, Kirksey A. Maternal body mass index and pregnancy consequence in the nutrition collaborative enquiry support program. Eur J Clin Nutr. 1994;48:S68–76.
-
Prentice AM, Goldberg GR, Prentice A. Body mass index and lactation performance. Eur J Clin Nutr. 1994;48:S78–86.
-
Roy SK. Factors affecting the piece of work productivity of Oraon agricultural laborers of Jalpaiguri district, Westward Bengal. Am J Phys Anthropol. 2002;117:228–35.
-
Stewart WF. RicciJA, Chee E. Morganstein D Lost productive work time costs from wellness weather condition in the United States: Results from the American productivity inspect Health and Lost Productive Fourth dimension in the U.s.a. Workforce. 2003;45:1234–46.
-
Thomas D, Frankenberg E. Wellness, nutrition and prosperity: a microeconomic perspective. Bull Earth Health Organ. 2002;lxxx:106–13.
-
Sachs JD, McArthur JW. The millennium projection: a plan for coming together the millennium development goals. Lancet. 2005;365:347–53.
-
McGregor SG, Cheung YB, Cueto South, Glewwe P, Richter L, Strupp B, et al. Developmental potential in the showtime 5 years for children in developing countries. Lancet. 2007;369:threescore–70.
-
FAO. The State of Food Insecurity in the World. 2004. http://www.fao.org/3/a-y5650e.pdf. Accessed 12 April 2018.
-
Salama P, Spieqel P, Talley L, Waldman R. Lessons learned from complex emergencies over by decade. Lancet. 2004;364:1801–13.
-
Immature H, Borrel A, Holland D, Salama P. Public diet in circuitous emergencies. Lancet. 2004;364:1899–909.
-
Bharati S, Pal 1000, Bhattacharya BN, Bharati P. Prevalence and causes of chronic energy deficiency and obesity in Indian women. Hum Biol. 2007;79:395–412.
-
Misra A, Pandey RM, Devi JR, Sharma R, Vikram NK, Khanna N. High prevalence of diabetes, obesity and dyslipidaemia in urban slum population in northern India. Int J Obes Relat Metab Disord. 2001;25:1722–nine.
-
Khongsdier R. Body mass index and morbidity in adult males of the war Khasi in Northeast Bharat. Eur J Clin Nutr. 2002;56:484–9.
-
Lee RD, Nieman DC. Nutritional Assessment. 3rd ed. Boston: McGraw Hill; 2003.
-
Shetty PS, James WPT. Body mass index. A measure of chronic free energy deficiency in adults. FAO Food Nutr Pap. 1994;56:i–57.
-
Norgan NG. Torso mass index and nutritional status: the effects of adjusting body mass index for the relative sitting height on estimates of the prevalence of chronic energy deficiency, overweight and obesity. Asia Pac J Clin Nutr. 1995;4:137–nine.
-
Norgan NG. Population differences in body composition in relation to the body mass alphabetize. Eur J Clin Nutr. 1994;48:s10–25.
-
Gallagher D, Visser Thousand, Sepulveda D, Pierson RN, Harris T, Heymsfield SB. How useful is body mass index for comparison of trunk fatness across age, sex, and ethnic groups? Am J Epidemiol. 1996;143:228–39.
-
Rolland-Cachera MF, Cole TJ, Sempe Chiliad, Tichet J, Rossignol C, Charraud A. Body mass index variations: centiles from birth to 87 years. Eur J Clin Nutr. 1991;45:13–21.
-
Woodruff BA, Duffield A. Anthropometric assessment of nutritional status in boyish populations in humanitarian emergencies. Eur J Clin Nutr. 2002;56:1108–18.
-
Barba C, Cavalli-Sforza T, Cutter J, Darnton-Hill I, Deurenberg P, Deurenberg-Yap M, et al. Appropriate body-mass index for Asian populations and its implications for policy and intervention strategies. Lancet. 2004;363:157–63.
-
Ferro-Luzzi A, James WPT. Adult malnutrition: simple assessment techniques for use in emergencies. Br J Nutr. 1996;75:3–10.
-
James W, Mascie-Taylor G, Norgan N, Bistrian B, Shetty P, Ferro-Luzzi A. The value of arm circumference measurements in assessing chronic energy deficiency in tertiary world adults. Eur J Clin Nutr. 1994;48:883–94.
-
Groot LCPGMD, Sette S, Zajkas G, Carbajal A, Cruzz JAA. Nutritional status: anthropometry. Eur J Clin Nutr. 1991;45:31–42.
-
Weiner JS, Lourie JA. Practical human biology. London: Academic Press; 1981.
-
Anderson FAD, Engledow JL. A simulation written report of methods for hypothesis testing in factor analysis. J Consum Res. 1980;7:141–50.
-
Klecka WR. Discriminant assay. California: Sage Publications; 1980.
-
Pal B, Seal B, Roy SK. Nutritional status of fishermen communities: validation of conventional methods with discriminant role analysis. Bulletin of Mathematical Sciences and Applications. 2014;8:49–59.
-
Perissinotto E, Pisent C, Sergi Grand, Grigoletto F, Enzi G. Anthropometric measurements in the elderly: age and gender differences. Br J Nutr. 2002;87:177–86.
-
Enzi Yard, Gasparo M, Biondetti PR, Fiore D, Semisa M, Zurlo F. Subcutaneous and visceral fatty distribution according to sex, age, and overweight, evaluated by computed tomography. Am J Clin Nutr. 1986;44:739–46.
-
Corish CA, Overflowing P, Kennedy NP. Comparison of nutritional risk screening tools in patients on access to hospital. J Hum Nutr Diet. 2004;17:133–ix.
-
Sanchez-Garcia S, Garcia-Peria C, Dugue-Lopez MX, Juarez-Cedillo T, Cortes-Nunez AR, Reyes-Beaman S. Anthropometric measures and nutritional status in a good for you elderly population. BMC Public Health. 2007;7:ii–9.
-
Burgert SL, Anderson CF. An evaluation of upper arm measurements used in nutritional assessment. Am J Clin Nutr. 1979;32:2136–42.
-
Falciglia G, O'Connor J, Gedling Eastward. Upper arm anthropometric norms in elderly white subjects. J Am Diet Assoc. 1988;88:569–74.
-
Kapoor Due south, Verma D, Bhasin P, Dhall M, Bharadwaj S. Skinfolds as prognosticators of nutritional status among adult undernourished males of Bharat. Int J Sociol Anthropol. 2012;4:257–65.
-
Banik SD. Health and nutritional status of three adult male populations of eastern India: an anthropometric appraisal. Italian Journal of Public Health. 2009;half-dozen:294–302.
-
De K. Written report nutritional status by waist circumference and waist hip ratio. Periodical of Health and Medical Informatics. 2017;8:248–ix.
-
Deurenberg P, Deurenberg-Tap One thousand, Guricci S. Asians are different from Caucasians and from each other in their trunk mass index/trunk fat percentage relationship. Obes Rev. 2002;3:141–6.
Acknowledgements
AB's enquiry is supported by Indian Statistical Institute.
Availability of information and material
The datasets used and/or analysed during the electric current study are available from the corresponding author on reasonable request.
Funding
The enquiry is a part of an ongoing bio-medical project funded past Indian Statistical Institute.
Author information
Affiliations
Contributions
SKR and AB participated in designing and coordination of the written report. AB contributed to data collection. AB and BP contributed to data analysis. AB drafted the manuscript. BP, SM and SKR contributed towards the critical revision on all versions of the manuscript. All authors read and approved the concluding manuscript.
Corresponding author
Ethics declarations
Ethics approval and consent to participate
Data were collected as role of an ongoing bio-medical project approved past the Ethical Committee of Indian Statistical Institute and was performed with the prior written consent of the participants.
Consent for publication
Non applicative.
Competing interests
The authors declare that they have no disharmonize of involvement.
Additional information
Publisher's Notation
Springer Nature remains neutral with regard to jurisdictional claims in published maps and institutional affiliations.
Rights and permissions
Open Access This article is distributed nether the terms of the Creative Commons Attribution four.0 International License (http://creativecommons.org/licenses/by/4.0/), which permits unrestricted use, distribution, and reproduction in any medium, provided you give appropriate credit to the original author(s) and the source, provide a link to the Creative Commons license, and indicate if changes were fabricated. The Artistic Eatables Public Domain Dedication waiver (http://creativecommons.org/publicdomain/nil/1.0/) applies to the data made available in this article, unless otherwise stated.
Reprints and Permissions
About this article
Cite this commodity
Bhattacharya, A., Pal, B., Mukherjee, S. et al. Assessment of nutritional status using anthropometric variables by multivariate assay. BMC Public Health 19, 1045 (2019). https://doi.org/10.1186/s12889-019-7372-two
-
Received:
-
Accepted:
-
Published:
-
DOI : https://doi.org/10.1186/s12889-019-7372-2
Keywords
- Nutritional status assessment
- Anthropometric measurements
- Confirmatory factor analysis
- Discriminant function analysis
Source: https://bmcpublichealth.biomedcentral.com/articles/10.1186/s12889-019-7372-2
0 Response to "2. what other tools might be used to assess mrs. baxter’s nutritional status?"
Post a Comment